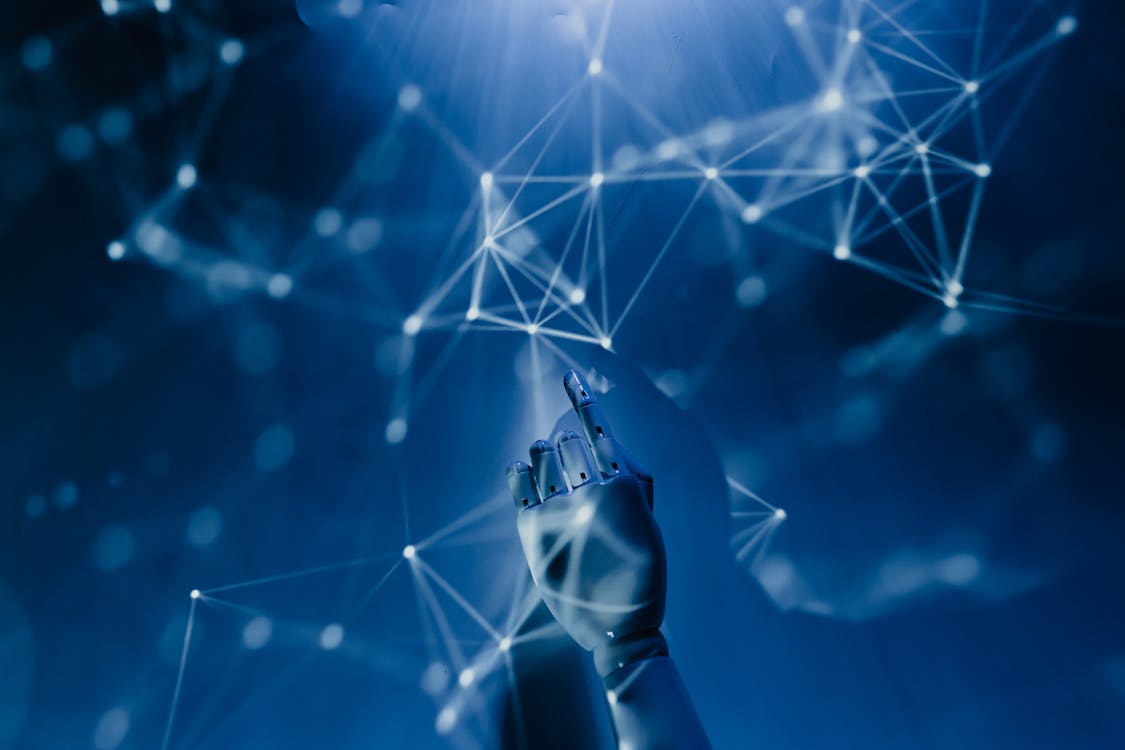
Deloitte's report aptly captures the essence of the current AI landscape: "Today's race transcends mere adoption of AI or process automation for efficiency. It's about harnessing AI's potential to create new opportunities for businesses, employees, and society. It's about breaking free from traditional business constraints." With such innovation, however, comes the need to address several security challenges.
Key difficulties in artificial intelligence development and implementation include inaccuracy (56%), cybersecurity concerns (53%), and risks of intellectual property infringement (46%). With 94% of enterprise leaders acknowledging AI's critical role in future success, understanding these problems and their solutions is crucial. In this context, we will explore seven such risks and various strategies to safeguard AI apps, ensuring they are secure, reliable, and aligned with business goals.
Data Quality and Quantity
In the realm of AI development, the challenge of data volume and credibility is paramount. High-quality, voluminous datasets are crucial for training robust models. One effective solution is employing data augmentation techniques, which artificially expand dataset size, enhancing the diversity and depth of material.
Rigorously cleaning and preprocessing information is also essential, ensuring accuracy and relevance in artificial intelligence self-learning. Implementing these methods not only improves the model's performance but also bolsters AI security, as well-processed data reduces the risk of biases and vulnerabilities in such systems. Such meticulous attention to data reliability is a cornerstone of professional and secure AI development.
Algorithm Bias and Fairness
Bias and hallucination in intelligent solutions development pose significant challenges, impacting both effectiveness and AI security. A key strategy to mitigate this is using varied training datasets. These repositories ensure representativeness and inclusivity, crucial for fair and unbiased outcomes.
Additionally, implementing fairness metrics and thorough testing during the model creation is essential. This practice helps identify and rectify any biases that might arise, ensuring the technology operates justly across various scenarios and demographics. Addressing this challenge not only enhances the reliability of AI systems but also fortifies their safety, making them more resilient to manipulation and misuse in diverse applications.
Model Explainability and Transparency
Ensuring accountability and openness is crucial for both user trust and AI security. To achieve this, it's important to document and sincerely communicate how AI models arrive at decisions. This transparency allows clients and stakeholders to gain insight into its functioning, fostering confidence. Additionally, providing comprehensive training and resources is key for customers to effectively interpret and utilize AI outputs. Such efforts not only demystify internal processes but also contribute to a secure artificial intelligence ecosystem, where users are well-informed and can detect potential anomalies or biases in the tool's behavior. These practices are fundamental in creating accountable and trustworthy technology.
Integration with Existing Systems
The integration of AI solutions into the current infrastructure demands strategic planning and execution. Developing the tools with modular and adaptable architectures is essential. This approach ensures that new components can seamlessly interface with pre-existing setups, a critical aspect that must be addressed by partners specializing in AI development.
Thorough compatibility and integration testing is another vital step, safeguarding against probable operational disruptions. Additionally, employing middleware solutions is a practical method for bridging artificial intelligence systems with legacy infrastructures. These elements not only smooth the process but also enhance AI security, acting as a safeguard between renewed and old architecture. Such meticulous implementation strategies are key to leveraging the full potential of the technology in existing business ecosystems.
Data Privacy and Security
Protecting sensitive information is paramount when it comes to AI-powered applications. Implementing end-to-end encryption for both data storage and transmission forms a strong defense against unauthorized access. This method ensures that info remains secure from the point of origin to its destination.
Regular updates to security protocols are equally important, keeping the AI systems resilient against evolving cyber threats. Additionally, conducting periodic vulnerability assessments helps identify and address potential weaknesses promptly. These proactive measures are fundamental in maintaining the integrity and confidentiality of data within intelligent solutions, safeguarding them against breaches and ensuring trust in internal processes.
Regulatory Compliance and Standards
Adhering to set norms and protocols is vital for AI security, especially in heavily regulated industries. Staying abreast of sector-specific regulations and compliance requirements is a continuous process, essential for ensuring AI solutions meet legal standards. Engaging with corresponding experts plays a crucial role in this procedure, as they provide the expertise needed to navigate the complex landscape and certify that applications are compliant.
Additionally, developing tools powered by artificial intelligence and with built-in checks and audits is a proactive approach. This not only streamlines the compliance strategy but also embeds a layer of protection, securing ongoing adherence to restrictions and safeguarding against potential breaches.
Scalability of AI Solutions
Ensuring the adaptability of AI-powered tools is key to their long-term viability and effectiveness. Designing AI systems with scalable architectures from the beginning is crucial. This foresight allows for easy expansion and adaptation as demands and data volumes increase.
Additionally, it's important to conduct regular reviews and updates of apps. These periodic revisions ensure that artificial intelligence can handle increased loads and more complex tasks without compromising performance. Such flexible design and continuous improvement are essential for maintaining efficient and robust AI infrastructures, capable of growing and evolving alongside the businesses they serve.
Takeaways
As we conclude our exploration, it's evident that the journey to harnessing AI's full potential is both challenging and rewarding. From ensuring data quality and quantity to addressing scalability, each challenge presents an opportunity to fortify the technology's reliability and security. Employing augmentation, advocating for algorithm fairness, securing model transparency, and integrating AI seamlessly into existing systems are not just solutions; they are essential steps toward creating AI that is not only powerful but also trustworthy and ethical.
Moreover, the emphasis on privacy, safety, regulatory compliance, and the ability to scale AI-powered tools underscores the multifaceted approach required in the development services. As artificial intelligence continues to evolve, these aspects will remain pivotal in guaranteeing that it serves as a catalyst for innovation and efficiency, rather than a source of vulnerability.
The implementation of AI is as much about overcoming technical hurdles as it is about adhering to standards and building trust. In this rapidly advancing field, staying vigilant, adaptable, and informed is key. By embracing these challenges, businesses can ensure that their AI initiatives succeed and set new benchmarks in security and efficacy.