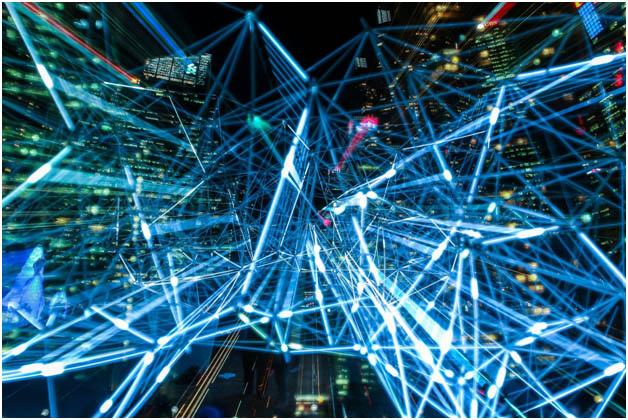
What do Netflix suggestions, driverless cars, chatbots, Facebook’s face recognition, and Apple’s (News - Alert) Siri all have in common? If you said that they all are “convenient technological advancements”, you are not wrong. However, to be more specific, the thing that binds them all together is that they all use one of the most impressive technological developments in the last years—deep learning.
Software development companies from all around the world have achieved the seemingly impossible: to replicate the brain’s inner workings through a combination of powerful algorithms. Thus, they are now able to embed devices, platforms, and applications with neural networks capable of analyzing data, providing insights, and coming up with their decisions based on their own logic.
So, what’s the deal with deep learning? Certainly a lot.
What’s deep learning, really?
Deep learning is a part of artificial intelligence (AI) that emulates the way the human brain works when processing data. In that way, it analyzes huge data sets and is able to create patterns with its own logic to later use them in decision making. Theorized back in the 80s, this advanced technology is only possible today thanks to the evolution in computing power and the massive amount of data available.
In fact, it’s impossible to think of deep learning appearing in a different context. That’s because there was no other time in human history where so much information was so readily available. Give thanks for that to the popularization of social media, search engines, e-commerce stores, and cloud-based platforms, among many others. All of those became sources of unstructured data that would take forever for humans to process and understand.
That’s why software development services resorted to AI to take advantage of that information and deep learning was born. This technology uses a hierarchical level of artificial neural networks to process data. In other words, deep learning employs several layers of self-adaptive algorithms to analyze information and identify patterns. Those layers work like neuro nodes connected with one another in a web-like form. Thus, each node is capable of processing data with different approaches, which allows for non-linear evaluation of all the information.
The difference between deep learning and machine learning
It’s highly important to understand that deep learning isn’t the same as machine learning, though a lot of people interchange them at will. That’s because deep learning is a subset of machine learning. For its part, machine learning consists of algorithms developed to get progressively better at a specific function and requires guidance. If, for instance, a machine learning app performs its function inaccurately, an engineer has to make adjustments to its basic logic. In deep learning, it's the neural network itself that defines whether its functions are inaccurate or not.
An example can help better understand the difference. While a machine learning software can adjust the temperature according to certain triggers (someone saying “it’s cold” or “it’s hot”), a deep learning algorithm could be triggered by more complex triggers (someone saying “the sun is coming up” could imply that the temperature is about to go up, so the algorithm would adjust the temperature accordingly).
Real applications for deep learning
All of the above can be summed up in a very simple manner - we’ve reached a point in history where software development providers are developing applications capable of solving complex problems like a human would do, but without human interaction. It’s exciting and somewhat frightening to imagine all the possibilities and to think that there are a lot of uses that we can’t even fathom today.
However, we do have a lot of examples of real applications for deep learning, which show the technology’s power and display its full potential. Some of them include:
- Virtual assistants: Siri, Alexa, Cortana and other popular virtual assistants are based on deep learning algorithms to function. Through neural networks, these applications are capable of understanding the user’s speech and the language humans use to interact with them. In that way, they can carry out the actions they are asked to and, when they can’t, they provide alternatives and suggestions that improve over time to better fit the user’s particular needs.
- Driverless vehicles: today’s self-driving cars, trucks, and drones are all the rage, even when most of them haven’t stepped out from the pilot phases yet. However, they are in quite an advanced stage of development, with deep learning at the very core of their workings. Thus, driverless vehicles use deep learning algorithms to understand their surroundings and react accordingly. From reading traffic signs to anticipate the movements and interact with other vehicles, pedestrians, and even unforeseen incidents, neural networks are the ones actually driving these vehicles.
- Chatbots: one of the most popular reasons why companies of all sizes are considering the outsourcing of software development is coming up with solutions that provide more efficient ways of dealing with their customers. Chatbots are a perfect example of that. Through them, businesses can offer a smart and helpful communication channel for leads and clients to find answers and solutions. As it happens with all deep learning algorithms, chatbots learn more the more interactions they have, so now it’s not surprising for them to be incredibly conversational, to the point where people can’t tell if they are being serviced by a human or a bot.
- Facial recognition: controversial as it stands right now, facial recognition is still being introduced in a multitude of services and applications. Probably the most renowned one is Facebook’s use of deep-learning-based recognition to tag (News - Alert) images uploaded to the platform. Naturally, this isn’t the only potential use. Some of the tests that are being conducted right now have facial recognition applications as part of security systems in public spaces. The purpose is to use neural networks to aid in the search of missing persons, while also quickly identify criminals and terrorists. In this case, deep learning is more than just the comparison of a face against a database, because the algorithms are capable of factoring in changes in hairstyles, minor surgeries, and even modifications due to the conditions of the place where the image is taken.
- Medical research: one of the most promising uses for deep learning is to be found in the medical research field, as neural networks are helping to search for new treatments and medicines to fight all kinds of diseases. One of the most investigated medical uses focuses on cancer and the early diagnosis of tumors in people that haven’t had any symptoms. Additionally, deep learning methods are being used to create personalized medicines designed for a specific individual’s genome. This would open the door for a wildly different approach to medicine, as treatments would be as unique as the patients being treated.
- Entertainment: we’ve mentioned Netflix before but there are several companies using deep learning algorithms to bring new features to their platforms. Netflix’s own recommendation engine uses them to understand the user likes and dislikes and provide more accurate content suggestions. Spotify (News - Alert) is working with this technology to come up with a way to adjust recommendations to a person’s mood. And Amazon is employing deep learning techniques to offer items based on similarity and convenience when compared with items in the user’s purchase history and previous searches.
The future of deep learning
Everything we’ve mentioned above is just the tip of the iceberg for deep learning. As the technology becomes more widely available and more people start to interest themselves in it, more companies and organizations will consider software development outsourcing to come up with their own uses for neural networks.
Given the ability of deep learning algorithms to tackle vast amounts of disparate data and the increasing availability of information from all kinds of sources, it would make sense for this technology to soon become an essential foundation for applications of all kinds. And that’s even truer when considering that deep learning becomes more efficient as more data is fed to it.
So, we’re on the verge of a major step in the so-called information society. As deep learning continues to evolve, we’ll start to make more sense of more data and we’ll get insights we could have never gotten before. The possibilities that can bring on real-life scenarios are certainly endless as, to be honest, the only limitation is how far we can take our imagination when it comes to deep learning.