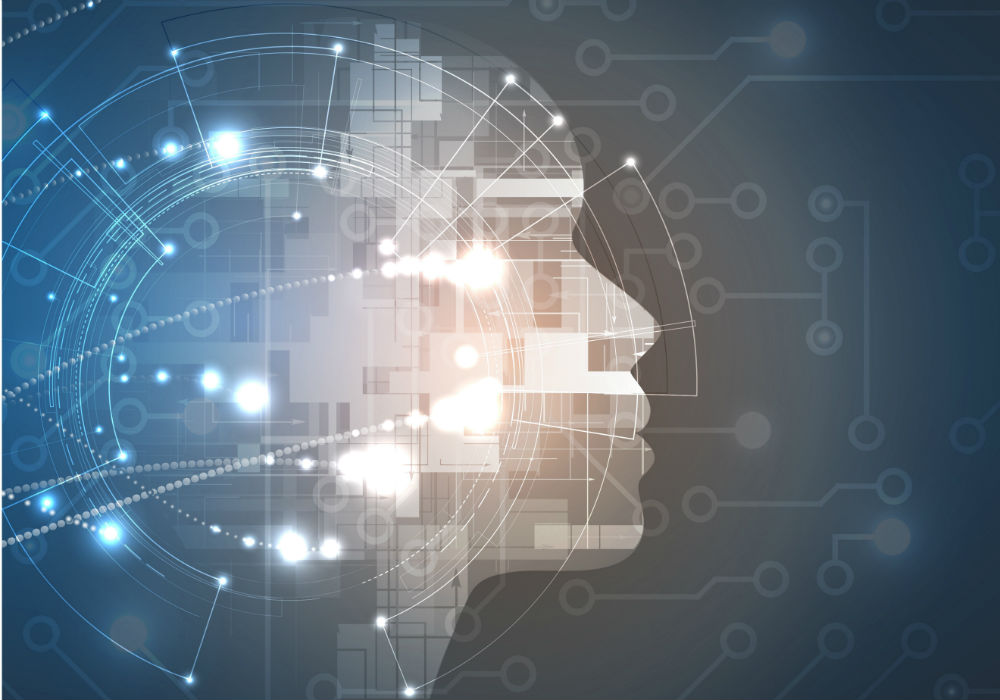
According to PricewaterhouseCoopers, more and more companies plan to invest in artificial intelligence in the next years. Indeed this technology is considered as a way to scientifically change most business processes.
Originated in the middle of XX century, the field of artificial intelligence combines a wide range of scientific fields: knowledge representation, machine learning, processing of big data, etc. Machine learning systems attract a lot of attention from computer programmers.
In contrast to the classical algorithmic methods, machine learning is not based on solving a specific problem, but on learning to solve similar problems and finding the subsequent solution of the specific problem. The spectrum of the methods used is extremely wide: optimization methods, mathematical statistics, probability theory, graph theory, and artificial neural networks.
Neural networks often come to the fore. Despite the fact that, like artificial intelligence in general, neural networks have been developing since the middle of the last century, only in recent years have they really become actively used. This is due to the large amounts of data available for processing and training of neural networks. At the same time, the advent of more powerful computers allows such data volumes to be processed quickly, primarily using graphics accelerators and neuromorphic processors like IBM (News - Alert) TrueNorth.
As with the use of any other technology related to data processing, the information security specialists are confronted with the question of possible threats and measures to counter such threats when using artificial intelligence systems.
In information security, the use of artificial intelligence is usually considered in the context of fighting existing cyberattacks, such as phishing, new types of malware, DDoS, etc. At the same time, such tools can provide a new level of running the previously mentioned attacks if AI is used by cybercriminals.
Like any other technology, the use of artificial intelligence is accompanied by a wide range of previously unknown threats that extend from social and ethical problems related to the restriction of civil liberties and pluralism of opinion in decision-making automation, as well as responsibility for their consequences. Other issues have to do with the security of technical implementation of artificial intelligence systems, and relating, for example, to ensuring confidence in the decision-making process or the security of the stored and processed data.
How to ensure AI ??systems security
The principle of preliminary learning noted above (when data gets processed by methods of artificial intelligence) leads to the fact that the final decision depends not only on the decision-making algorithm but also on the previously and currently processed data. As a result, there are two completely new types of attacks on the systems of this type in addition to the classical ones typical of any information system:
- Manipulation of input data during learning\training in order to change the subsequent decision-making process, or the so-called Data Poisoning.
- Selection of wrong input data at the decision-making stage, leading to their incorrect classification, or the so-called Data Evasion.
These and other attacks are applicable not only to neural networks but also to machine learning methods that use, for example, the apparatus of mathematical statistics. This is a consequence of the fact that all such methods actually approximate the parameters of the data being processed by some functional relationships. Actually, the accuracy of such an approximation determines the possibility of implementing this type of attacks.
It should be noted that at present there is no universal methodology for protecting AI systems. However, researchers have identified a number of approaches, which are currently being actively studied.
Protection against attacks on the decision-making process
In this case, the training data is formed in such a way as to exclude the possibility of using any possible attacks. In fact, systems learn to recognize the rogue data (presented by attackers) thus trying to weaken the influence of certain classes of attacks.
The idea of ??protection integrated into the learning process is based on limiting the set of input data. As already mentioned, the data parameters can have a complex functional form, and the decision-making rule actually approximates them with a simpler form. So, for example, a ban on the use of data that is in some sense far from the average value of the training sample reduces the impact of attacks at the training stage.
In conclusion it is important to note that working with AI systems, it very crucial to ensure the security of input data and in particular the security of personal data of users. Processing large amounts of data by machine learning systems is undoubtedly endangering private user data in the first place. At present, attempts are being made to combine systems of this class with such actively developing and promising areas of cryptography, such as homomorphic encryption and secure multi-party computation protocols. However, we are still far from real implementation of such systems.